Welcome
What is Parea AI?
Parea helps AI Engineers build reliable, production-ready LLM applications. Parea provides tools for debugging, testing, evaluating, and monitoring LLM-powered applications.
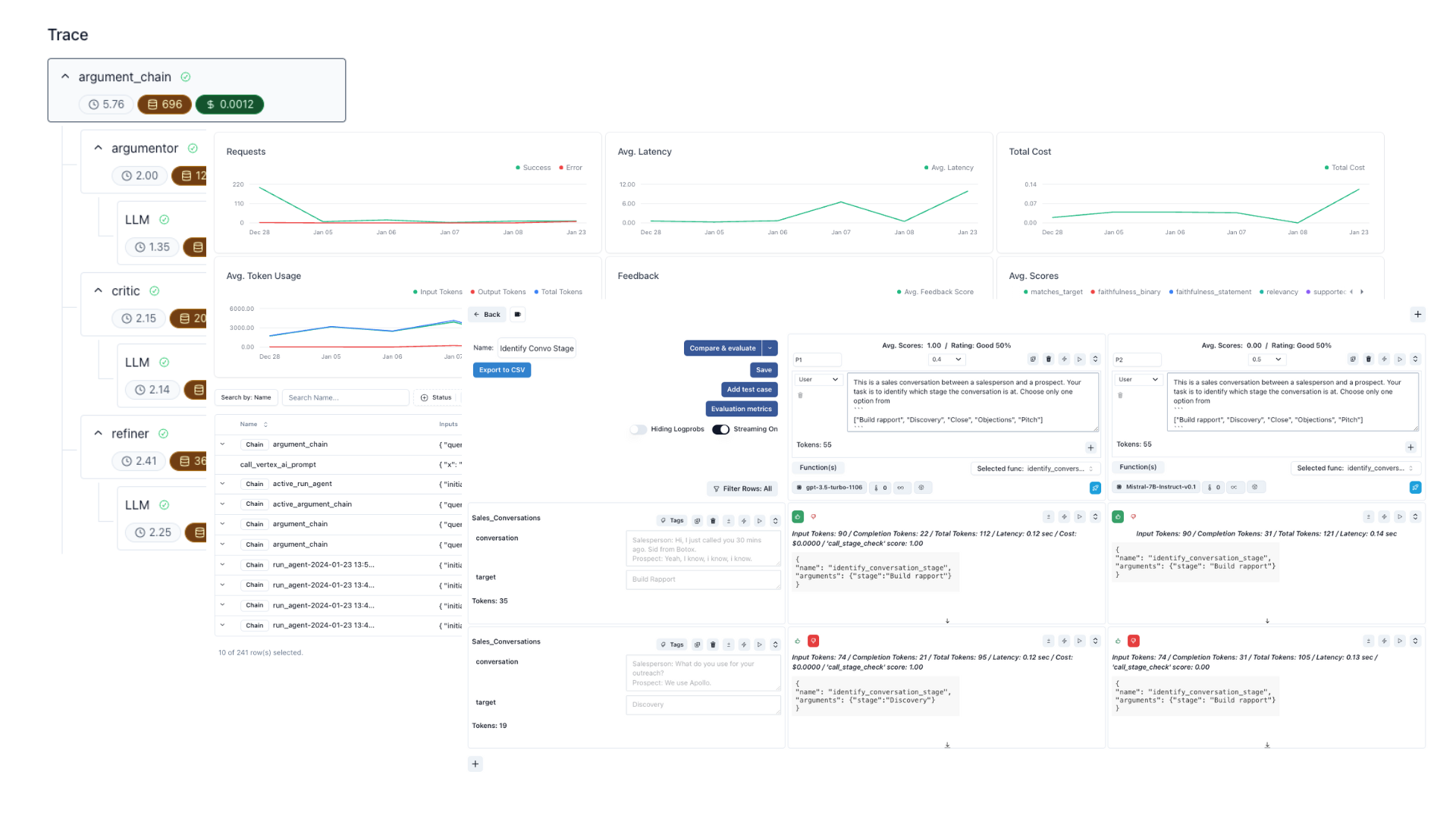
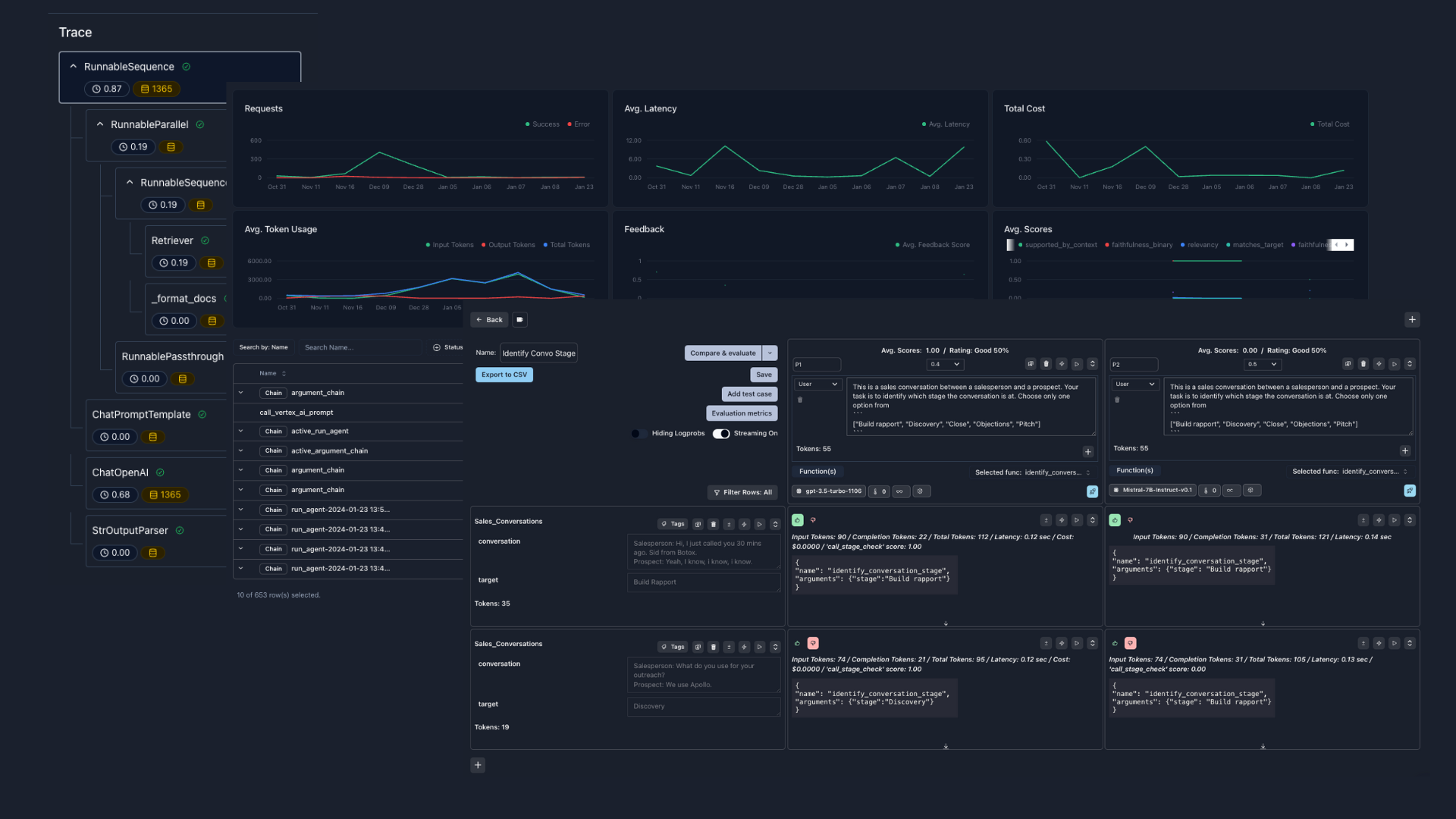
Getting Started
It takes less than 2 minutes and only 2-3 lines of code to integrate Parea into your LLM stack.
Evaluation
Start evaluating your LLM app
Monitoring
Start monitoring your LLM API calls
Tutorial
Use Parea to improve a RAG application
Features
A feature for every step of your application lifecycle.
Evaluation & Testing
Evaluate your LLM app and track metrics
Observability
Capture key metrics, LLM request and responses, and custom metadata for your entire application
Prompt Playground
Grid-style playground to iterate on prompts
Getting Help
If you have any questions, need help, or have feedback, there are many ways to contact us:
Was this page helpful?